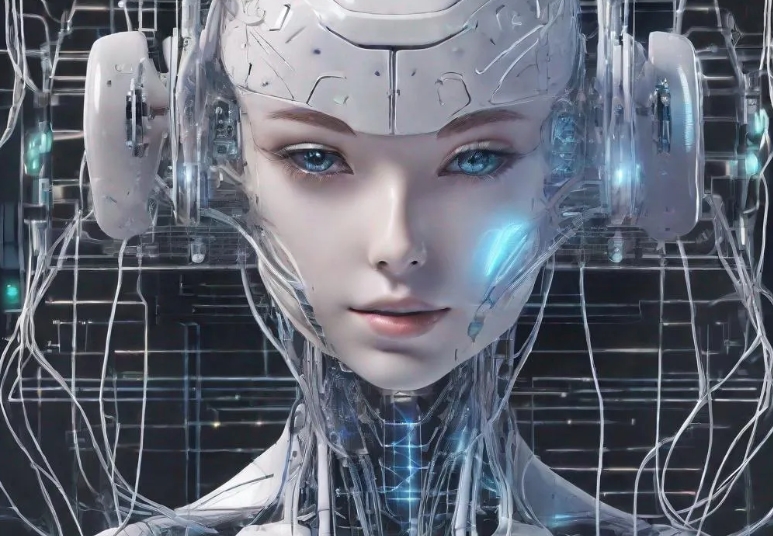
Ready to dive into machine learning? This guide breaks down the essentials to get you started with AI and data science. Unlock the potential of ML and transform your skills!
1. Understand the Basics
Start by familiarizing yourself with key concepts:
Types of Machine Learning:
- Supervised Learning
- Unsupervised Learning
- Reinforcement Learning
Common ML Algorithms:
- Linear Regression
- Logistic Regression
- Decision Trees
- Neural Networks
Key Terms:
- Training Data vs. Test Data
- Overfitting and Underfitting
- Bias and Variance
2. Build a Strong Foundation in Mathematics
Focus on these mathematical areas:
- Linear Algebra
- Calculus
- Probability and Statistics
Resources: - Khan Academy (free online courses) - "Mathematics for Machine Learning" by Marc Peter Deisenroth (book)
3. Learn a Programming Language
Popular languages for ML:
Python:
- Most widely used in ML and data science
- Libraries: NumPy, Pandas, Scikit-learn
R:
- Specialized for statistical computing
- Useful for data analysis and visualization
Julia:
- Growing in popularity for its speed and ease of use
Recommendation: Start with Python due to its versatility and extensive ML libraries.
4. Master Data Preprocessing
Learn essential data handling skills:
- Data Cleaning
- Feature Selection and Engineering
- Data Normalization and Standardization
Tools: - Pandas for data manipulation - Matplotlib and Seaborn for data visualization
5. Start with Simple Projects
Begin with beginner-friendly projects:
- Iris Flower Classification
- Handwritten Digit Recognition (MNIST dataset)
- House Price Prediction
These projects will help you apply basic ML algorithms and understand the workflow.
6. Explore ML Frameworks and Libraries
Familiarize yourself with popular ML tools:
- Scikit-learn: For traditional ML algorithms
- TensorFlow and PyTorch: For deep learning
- Keras: High-level neural network API
7. Take Online Courses
Enroll in structured learning programs:
- Coursera: "Machine Learning" by Andrew Ng
- edX: "Machine Learning" by Columbia University
- Fast.ai: Practical Deep Learning for Coders
8. Read Books and Research Papers
Expand your knowledge with literature:
- "Hands-On Machine Learning with Scikit-Learn and TensorFlow" by Aurélien Géron
- "Deep Learning" by Ian Goodfellow, Yoshua Bengio, and Aaron Courville
- ArXiv.org for latest research papers
9. Join ML Communities
Engage with other learners and professionals:
- Kaggle: Participate in competitions and discussions
- GitHub: Contribute to open-source ML projects
- Stack Overflow: Ask questions and help others
- Local Meetups: Attend ML-focused events in your area
10. Practice Regularly
Consistent practice is key to mastering ML:
- Solve coding challenges on platforms like LeetCode
- Work on personal projects to apply your skills
- Participate in Kaggle competitions
11. Specialize in an Area of Interest
As you progress, focus on specific domains:
- Computer Vision
- Natural Language Processing
- Reinforcement Learning
- Time Series Analysis
12. Stay Updated
Keep up with the rapidly evolving field:
- Follow ML researchers and practitioners on social media
- Attend conferences (virtually or in-person)
- Read ML blogs and newsletters
Remember, learning machine learning is a journey that requires patience and persistence. Start with the basics, build a strong foundation, and gradually tackle more complex concepts and projects. With dedication and practice, you'll be well on your way to becoming proficient in this exciting and rapidly evolving field.
american-boffin.com
bfbchamp.com
democraticcoma.com
tigrepelvar.com
charpoles.com
derbywheelblazers.com
fansfocus.net
guildnow.com
hediyeteyze.com
isprimecdn.com
kiira-korpi.net
manutd24.com
mediumtylerhenry.com
mishanghai.org
savethreestrikes.com
smilesbydavis.com
10puntos.net
band-shirt.com
icelandtrails.com
paulmarioday.com
thefunnynanny.com
Dave Tries Ballet
Buon Grande
Criacao Sites
Perry Perkins Books
Writing Essay in AU
Ka Soku
Blood is Blood Movie
Eleanor Writes Things
The Happy Prince Beirut
Town of Witless Bay
Online Igrovoi Club
Trigeminal Neuralgia - Ronald Brisman MD
Chocolate City Burlesque
Advanced Electric Scooters
W Tougei
Breadboard Maniac
Takasu App